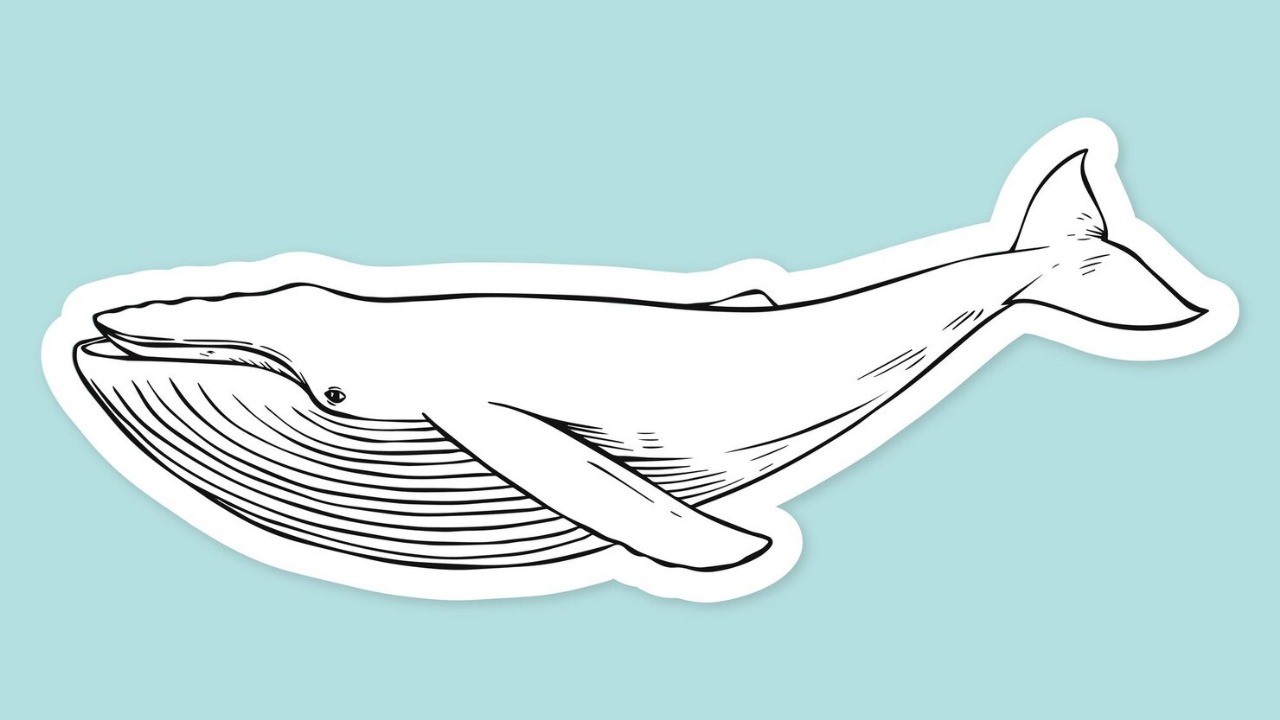
Artificial Intelligence and Competition: Navigating Uncharted Waters
Artificial Intelligence and Competition: Navigating Uncharted Waters
*This is an AI-powered machine translation of the original text in Portuguese
**Coauthored with Marco Almada. Originally published in Conjur.
*** Image resource obtained from Freepik.com
Debates about the intersection of artificial intelligence and competition have focused, on one hand, on the application of AI as a tool for investigations (computational antitrust) [1], and on the other hand, on the possibility of algorithmic collusion [2]. Although some studies point to the possibility of tacit collusion in experimental scenarios [3], others indicate that AI systems would not be sufficient to address the typical instability problems associated with collusion among humans [4]. In fact, no conclusive cases of algorithmic collusion have been detected [5], which is why Jérôme de Cooman refers to the topic as the "white whale of competition law" [6]: exhaustively sought but never found.
While discussions on AI ethics often reference concerns about potential market concentration, few works thoroughly explore the problem using a competition analysis methodology [7]. Therefore, it seems appropriate, in this field, to shine a light not only on the impacts of using intelligence on competition or enforcement but also on the competitive conditions in emerging and growing AI markets.
This attention makes sense when we realize that AI systems, especially for more complex machine learning methodologies, require vast amounts of data, highly specialized experts, increasingly scarce in the market, and large-scale computing resources [8], the implementation of which can be quite costly.
For example, it is estimated that the training costs for the GPT-4 language model exceed $100 million [9]. In the current state of technology, especially when we talk about foundational models, few market players have access to the technical, human, and financial resources needed to create their own state-of-the-art AI systems [10].
As a result of these requirements, AI markets tend to concentrate on a few dominant players. The leading providers have sufficient human and financial resources to become major investors in research and development and to capture synergies with downstream markets [11], conditions that collectively bolster their position and competitiveness, resulting in a potential "moat" to be crossed by new entrants [12].
The vast majority of developers do not create their own technologies but depend on AI-as-a-service solutions in which large-scale technical providers offer pre-trained models to be adjusted for specific applications. In this way, providers of large AI models become the heart of a digital ecosystem, with the orchestrator in the center and service-dependent agents as orchestrated complementors on the periphery.
In the same AI ecosystem, orchestrated entities engage in a series of collaborative and competitive relationships. Two or more orchestrated entities may be in direct competition if they offer comparable products or services, as would be the case for two companies using GPT-4 to offer an automated contract generation service.
On the other hand, collaboration can take various forms depending on the synergies between the services offered. For example, automated generation services could benefit from potential integration with another orchestrator using GPT-4 to extract relevant information from existing contracts. Regardless of their relationship with each other, orchestrators are dependent on the ecosystem orchestrator, using the technology or services made available and subject to the terms defined by the orchestrator.
There is also competition between ecosystems, focusing on the competition between major players offering different value propositions. For example, the competition between the Android and iOS operating system ecosystems or the Kindle and iPad e-book ecosystems. Using this perspective to examine AI markets, we can anticipate some challenges for competition analysis.
First, it is necessary to characterize the competition dynamics among participants in AI markets, but the traditional definition of a relevant market can be complex, given that AI systems designed for different tasks may overlap in some functions, and there are general-purpose AI systems used in various markets with distinct applications. Additionally, AI systems can foster different downstream services, making it difficult to distinguish between an AI market and a market for an AI-powered service.
Other issues observed in competitive dynamics of ecosystems can be pointed out, such as lock-in effects. There is a learning and implementation cost associated with AI solutions for orchestrated entities, so migrating to a new ecosystem requires a complete adjustment of operational procedures to the technical specifics of the new AI technologies [13].
Protection of AI solutions through trade secrets can also act as a barrier to mobility and competitive dynamics [14], suggesting the need for regulation with requirements for interoperability [15], the impacts of which, however, still need further study. Another concern is the extent to which data-driven network effects can affect AI markets, with a feedback loop between providing the best solution and collecting larger volumes and varieties of data [16].
On the other hand, it is debatable whether concentration factors are actually an inevitable consequence of current AI technologies. Some arguments suggest that it is, in fact, a contingent aspect [17], considering that there is rivalry among major providers of large-scale AI models, and the launch of open-source models like Meta's LLaMA or Hugging Face's Bloom may create more room for rivalry. Large-scale models offered by established market agents have also prompted some degree of technological experimentation, indicating that relatively small models can be quite successful and competitive, at least for certain tasks [18].
What we currently see is developers racing to create increasingly larger models, which may point to a future oligopolized landscape.
However, the attachment to ex-ante regulation, such as imposing interoperability, perhaps driven by concerns about this scenario, comes precisely from ethical and regulatory approaches or industrial policy [19] that do not explore the potential of antitrust analysis tools as a means of ex-post resolution, as a way to ensure the contestability of AI markets, either through prior control of economic concentrations or through the repression of conduct.
The characterization of AI technology markets is essential to understand whether the practices of actors in AI markets negatively affect consumer surplus [20], and if so, whether these adverse impacts require a response from antitrust authorities [21], or conversely, whether this market necessitates preventive regulation [22].
This is a new field to be explored by experts and scholars, both legal and economic, to build knowledge to support the actions of competition authorities, but it cannot do without an understanding of the technical specifics of AI, which play a triple role in competition law.
First, it allows differentiating to what extent technical aspects consist of competitive advantages or true barriers to entry. Second, it allows understanding how technical choices within AI models can affect competition in downstream markets. Third, it enables the identification of potential hybrid dynamics that may go beyond the dimensions of intra and inter-ecosystem competition: for example, the development of open-source models and experimentation based on them can lead to scenarios where ecosystems are not entirely closed but admit a certain degree of integration among their constituents, giving rise to forms of competition across ecosystems, with an orchestrator differentiating itself through external solutions to compete within its ecosystem while simultaneously reinforcing competition among rival ecosystems (depending on the contractual relationships that begin to structure these markets).
It is important to remember that, with the evolution of AI in economic relations, knowledge about antitrust analysis in AI markets may be crucial in the future to understand the competition dynamics of any sector of the economy. Whether because AI becomes integrated into a range of products or because AI is ubiquitously used in the analysis and strategic decision-making by market agents. Therefore, the problems and conceptual tools of competition in AI markets will no longer be limited to technology markets.
In this scenario, the potential risks associated with algorithmic collusion are only a small part of the challenges and opportunities that AI brings to competition law. Therefore, rather than hunting for the white whale, it is better to learn to navigate the uncharted waters of AI markets.
[1] In this context: Lena Hornkohl, "Article 102 TFEU, Equal Treatment and Discrimination after Google Shopping" (2022) 13 Journal of European Competition Law & Practice.
Juliano Maranhão, Miguel Garzeri Freire, and Marco Almada, "Os Limites Da Concorrência Frente à Inteligência Artificial" in Paula Farani de Azevedo and Nicolo Zingales (eds), A aplicação do direito antitruste em ecossistemas digitais: desafios e propostas (FGV Direito Rio 2022).
Schrepel, Thibault and Groza, Teodora, The Adoption of Computational Antitrust by Agencies: 2nd Annual Report (June 15, 2023). 3 Stanford Computational Antitrust 55 (2023), Available at SSRN: https://ssrn.com/abstract=4476321.
Schrepel, Thibault, The Making of An Antitrust API: Proof of Concept (October 12, 2022). Stanford University CodeX Research Paper Series 2022 3 Stan. Computational Antitrust 22 (2023), Available at SSRN: https://ssrn.com/abstract=4241927.
Schrepel, Thibault, Computational Antitrust: An Introduction and Research Agenda (January 15, 2021). Stanford Computational Antitrust (Vol. 1) 2021, Available at SSRN: https://ssrn.com/abstract=3766960.
[2] See, for example, Ezrachi and Stucke (n. 9); Nicolas Petit, "Antitrust and Artificial Intelligence: A Research Agenda" (2017) 8 Journal of European Competition Law & Practice 361; Antonio Capobianco and Pedro Gonzaga, "Competition Challenges of Big Data: Algorithmic Collusion, Personalized Pricing, and Privacy," Legal Challenges of Big Data (Edward Elgar 2020).
[3] E. Calvano, G. Calzolari, V. Denicoló et al., Algorithmic collusion with imperfect monitoring, International Journal of Industrial Organization, https://doi.org/10.1016/j.ijindorg.2021.102712.
[4] Thibault Schrepel, "The Fundamental Unimportance of Algorithmic Collusion for Antitrust Law" (Harvard JOLT Digest, July 2, 2020) https://jolt.law.harvard.edu/digest/the-fundamental-unimportance-of-algorithmic-collusion-for-antitrust-law accessed on September 24, 2021.
[5] Capobianco and Westrik (n. 76), paragraph 45.
[6] Jerome De Cooman, "Outsmarting Pac-Man with Artificial Intelligence, or Why AI-Driven Cartel Screening Is Not a Silver Bullet" (2023). 14 Journal of European Competition Law & Practice 186.
[7] An exception that proves the rule is the recent report from the UK Competition and Markets Authority on so-called "foundation models": https://www.gov.uk/government/publications/ai-foundation-models-initial-report.
[8] For example, massive cloud storage and machines with powerful GPUs and large memory: Andrew Lohn and Micah Musser, "AI and Compute: How Much Longer Can Computing Power Drive Artificial Intelligence Progress?" (2022); Neil C. Thompson and others, "The Computational Limits of Deep Learning" (arXiv, July 27, 2022) http://arxiv.org/abs/2007.05558 accessed on July 26, 2023.
[9] Will Knight, 'OpenAI's CEO Says the Age of Giant AI Models Is Already Over' [2023] Wired. https://www.wired.com/story/openai-ceo-sam-altman-the-age-of-giant-ai-models-is-already-over/ accessed on July 26, 2023.
[10] In fact, current metrics show a considerable level of concentration in both research and development and sector investments: Nestor Maslej and others, 'The AI Index 2023 Annual Report' (2023).
[11] For example, Meta and Amazon are the leading providers of AI technologies and, at the same time, use these technologies to power their internal functionalities, such as automated content moderation or e-commerce product recommendations.
[12] On the constituent elements of a moat, see Peter Thiel and Blake Masters, Zero to One: Notes on Startups, or How to Build the Future (Virgin Books 2014) 47-53.
[13] Cobbe, Jennifer, and Singh, Jatinder, Artificial Intelligence as a Service: Legal Responsibilities, Liabilities, and Policy Challenges (April 12, 2021). Jennifer Cobbe and Jatinder Singh 'Artificial Intelligence as a Service: Legal Responsibilities, Liabilities, and Policy Challenges' (2021). Computer Law & Security Review, 42, Available at SSRN: https://ssrn.com/abstract=3824736 or http://dx.doi.org/10.2139/ssrn.382473619.
[14] See, among others, Katarina Foss-Solbrekk and Ann Kristin Glenster, "The Intersection of Data Protection Rights and Trade Secret Privileges in "Algorithmic Transparency" in Eleni Kosta and Ronald Leenes (eds), Research Handbook on EU Data Protection Law (Edward Elgar Publishing 2022); Kristina Irion, "Algorithms Off-Limits? If Digital Trade Law Restricts Access to Source Code of Software Then Accountability Will Suffer," 2022 ACM Conference on Fairness, Accountability, and Transparency (Association for Computing Machinery, 2022).
[15] See, for example, debates about the value of data interoperability for competition: Oscar Borgogno and Giuseppe Colangelo, 'Data Sharing and Interoperability: Fostering Innovation and Competition through APIs' (2019) 35 Computer Law & Security Review 105314.
[16] OECD, 'Data Portability, Interoperability, and Digital Platform Competition' (2021) Discussion Document.
[17] Thibault Schrepel and Alex 'Sandy' Pentland, 'Competition between AI Foundation Models: Dynamics and Policy Recommendations' (2023). MIT Connection Science Working Paper 1-2023 https://papers.ssrn.com/abstract=4493900 accessed on August 4, 2023.
[18] Emma Roth, 'That Google Memo about Having "No Moat" in AI Was Real - and Google's AI Boss Disagrees with It' [2023] The Verge https://www.theverge.com/2023/7/10/23790132/google-memo-moat-ai-leak-demis-hassabis accessed on July 26, 2023.
[19] Josélia Elvira Teixeira and Ana Teresa CP Tavares-Lehmann, 'Industry 4.0 in the European Union: Policies and National Strategies" (2022) 180 Technological Forecasting and Social Change 121664.
[20] Or any other standard one may want to apply to assess competitiveness.
[21] See, among others, Nicolas Petit, Big Tech and the Digital Economy: The Oligopoly Scenario (Oxford University Press 2020).
[22] Schrepel, Thibault and Pentland, Alex, Competition between AI Foundation Models: Dynamics and Policy Recommendations (June 28, 2023). MIT Connection Science Working Paper 1-2003, Available at SSRN: https://ssrn.com/abstract=4493900 15-22.
Related publications
-
Data of Children and Adolescents in the Digital Environment
-
The National Data Protection Authority Applies Its Third Sanction
-
Artificial Intelligence in Fashion: Representations and Applications
-
Regulation of Artificial Intelligence in Future Jurisprudence: Civil Liability in Autonomous Vehicle Accident Cases